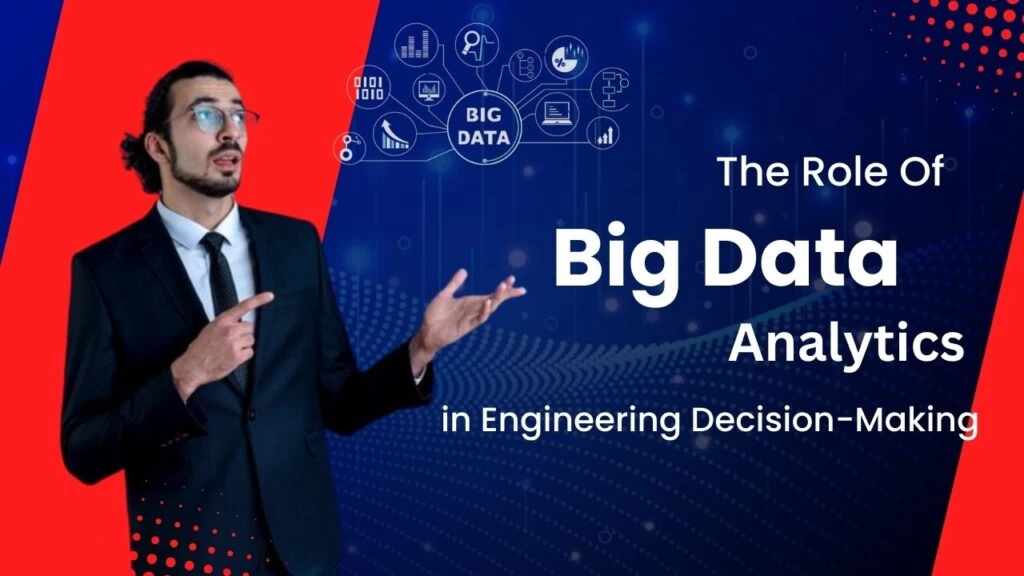
The integration of data and technology in modern engineering has created unprecedented opportunities for innovation. Big Data Analytics has emerged as a revolutionary force among the most remarkable developments, changing engineering domain decision-making. This article explains Big Data Analytics’ many facets and how it optimizes operations and improves project outcomes by giving us valuable insight into the sophistication of engineering processes.
Understanding Big Data Analytics
Big Data Analytics essentially involves the meticulous analysis of enormous and complex datasets to identify pertinent patterns, associations, and insights। In engineering, this means fully informing decision-making processes by using the massive amount of data generated throughout a project’s lifecycle। Bringing Big Data Analytics into engineering decision-making represents a departure from traditional approaches; a data-driven methodology improves the efficiency and effectiveness of various aspects of project management.
Optimizing Operations
Big Data Analytics’ capacity to optimize operations is a big part of engineering decision-making। Engineering projects can overwhelm traditional decision-making processes due to the sheer volume and complexity of the data that is generated। Big Data Analytics, on the other hand, helps to simplify this complexity by identifying gaps, weaknesses, and areas where improvement is possible। Engineers can make quick adjustments to ensure that operations run seamlessly and efficiently because they have real-time tracking of equipment performance, resource utilization, and project progress.
Predictive Analytics for Improved Planning
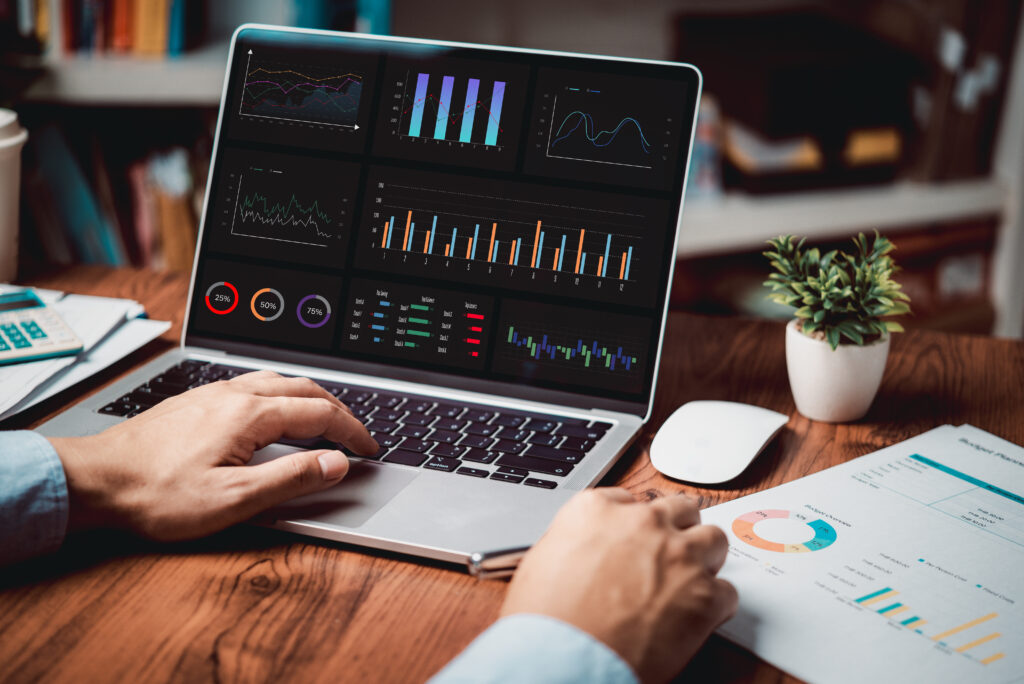
Predictive analytics, a capability that revolutionizes the way engineering projects are planned and executed, is made possible by big data analytics। Predictive models can predict potential problems and issues by using historical project data and considering several parameters । With this foresight, engineers can take a proactive approach and make informed decisions that anticipate and mitigate risks। As a result, Big Data integration of predictive analytics allows project managers to reduce delays, cut costs, and increase the likelihood of project success.
Enhanced Risk Management
Big Data Analytics becomes a critical ally in effective risk management, which is a critical component of successful engineering projects। Engineers can identify risk factors and quantify their potential impact by analyzing huge datasets related to past project performance, external variables, and stakeholder inputs। When used with this data-driven approach to risk management, it is possible to create strong mitigation strategies and make sure that decision-makers are informed, which leads to a more robust and adaptable project management framework.
Improved Resource Allocation
Resource management is a key factor in project success in engineering, and big data analytics is essential to maximizing resource usage. By closely examining resource availability, utilization, and related expenses, decision-makers can obtain a detailed grasp of resource dynamics. This realization makes it possible to allocate resources more effectively, avoiding overruns, maximizing utilization, and guaranteeing that every aspect of the project gets the assistance it needs. Big Data analytics essentially turns the process of allocating resources from one that is based on judgment and experience into one that is driven by data.
Real-Time Monitoring and Decision-Making
Because engineering projects are dynamic, it is imperative to monitor them in real time to maintain flexibility and reactivity to changing situations. This is made easier by big data analytics, which tracks project factors continuously and produces information as it happens. Decision-makers are better able to react quickly to changing conditions, make wise choices, and conduct initiatives properly when they have access to real-time data. This flexibility is significant because it enables engineering teams to quickly adjust and keep projects moving forward when unanticipated opportunities or obstacles present themselves.
Challenges and Considerations
Engineering decision-making greatly benefits from Big Data Analytics, but there are drawbacks as well. Obstacles that need to be overcome include data security issues, privacy concerns, and the requirement for qualified personnel who are adept at both data analytics and engineering. To make sure that ethical issues and best practices are incorporated into the decision-making processes, a concentrated effort is needed as the sector continues to embrace big data analytics.
Conclusion
To sum up, the incorporation of Big Data Analytics into engineering decision-making procedures represents a significant turning point with great potential for the sector. Big Data analytics is becoming an essential tool for guaranteeing the success of engineering projects since it may optimize operations, enable predictive analytics, improve risk management, and improve resource allocation. Embracing and utilizing the potential of big data analytics will be essential to keeping ahead in this cutthroat and always-evolving industry of engineering as it continues to change. The era of data-driven engineering decision-making is upon us, offering the prospect of more effective, knowledgeable, and prosperous projects.